Anna Wójcik: With “cultural analytics”, the big data craze has entered the humanities and social sciences. What is your contribution to this new field?
Lev Manovich: I introduced the term “cultural analytics” in 2005 to name something I knew was coming. Now it is here, it is big, and it is used in many contexts. Cultural analytics refers to the possibility of studying vast cultural content using computer tools. It was made possible thanks to two phenomena. First, we have gained access to more cultural content than ever before. Second, in addition to traditional tools used in the humanities and social sciences, we analyse this content with computer tools.
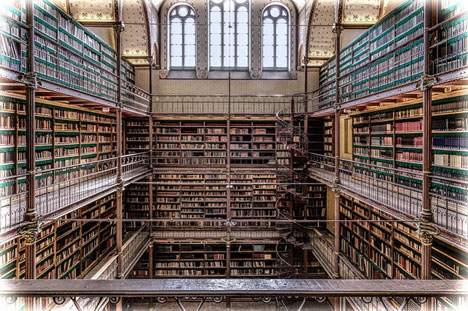
Rijksmuseum library, Amsterdam. Photo: Ton Nolles. Source:Flickr
There are two main sources of digital cultural content. The first is social media. The second comes from the digitization of historical content, which begun in the 1990s, and has reached a certain point now: for the first time, we have access to massive digital cultural datasets. Just imagine that the Rijksmuseum alone makes 250,000 images from their collections available to everyone online. Now we can analyse all of them at once. In my own lab we use big data to question how we think about culture, how we study culture, and how we classify culture, what we include and exclude as culture. In this way we challenge the humanities.
Is this approach more inclusive than those we had before?
The point is not to study big cultural data, meaning big in number, but to study more inclusive data sets. Let’s suppose I am interested in mid-twentieth-century Polish literature. The traditional method is to pick up between 20 and 25 authors who are considered prominent and go through their work. The other approach allows for analysis of all writings of all authors who published at that time. This means we are searching for general trends or connections that were previously overlooked.
To give an example, in my lab we have analysed Van Gogh’s paintings. There are digital images of around 800 van Gogh’s paintings available, and they represent 75 per cent of his body of work. Of course you could have analysed each painting one at a time, browsing traditional catalogues. But this old method makes noticing patterns more difficult. When you compare digitized images using analytics, you instantly discover new correlations and trends.
Computers are responsible for the data analysis, but – at least for now – the task of interpretation still belongs to human scientists. What is most difficult about interpreting cultural data sets?
It is very tempting to look at culture through familiar lenses and well-established categories: important/not important, male/female, white/non-white, city/country. Computer science tools give us the possibility to go beyond safe categories and safe questions in our research.
The traditional approach to research in the social sciences is to start with a research question and then go through documents or data. But when you allow the computer to go through and analyse all the novels and short stories by a certain Polish author, you may end up with several thousand clusters of similar words or phrases. This may change the way you formulate your research question.
Imagine that your goal is to investigate a newly discovered planet. What would you do first? Would you start with a question about whether the planet has oil or mountains? Or is it better to send a discovery mission to the planet to photograph its landscape, to get the picture? My advice is not to start with a research question, but to map what is where first. When we proceed like this, we get different results and end up with interpretations that vary from what we already know about our culture. This is our contribution to cultural development.
How does the content we post on the Internet reflect what society
actually thinks, if at all? Especially in the age of trolling and information wars?
Can we really say who society is? There are seven billion people in the world. A painting, a vase or a novel may hold a different set of moral and political values for every individual. Societies do not think. Individuals do. The real challenge of studying culture and society is to substitute generalizations, such as “Instagram is about fetishism” or “America is about capitalism” with more complex, nuanced concepts and relations.
Obviously, you cannot take social media content at face value. When you take a look at Instagram selfies taken in LA or Warsaw, everyone is smiling. But when you actually go to those cities you see that people are smiling more frequently in California than in Poland.
As concerns trolling, of course there is a certain number of fake accounts, but you can use algorithms to sort them out. The more important thing is that people don’t say what they think in social media. They create online personas. I imagine that we can introduce sophisticated algorithms, which would analyse the expressions of those personas and distinguish real meanings, opinions and motivations behind the surface of what is published online.
Big data becomes bad data when it threats our privacy. What measures should be taken by governments to guarantee their citizens basic digital rights?
Europe is more advanced in the discussion on privacy than other parts of the world. In the United States, websites do not ask your permission to use cookies or track your data. They just proceed. We need more strict laws about companies’ use of data. Business will oppose them, but they are absolutely necessary. And then we need to export this progressive approach all over the world, also to the United States.
Our eagerness to use scientific tools to analyse culture contrasts with twentieth-century critiques of technology. Is positivism back for good?
Good you mentioned positivism. In my course syllabus I have a lecture on the emergence of proper science, statistics and the idea of social physics. “Social physics” is a term introduced in the nineteenth century by August Comte, and revisited to a great fanfare in 2014 in Alex Petland’s book Social Physics: How Good Ideas Spread. This book promotes the idea that big data collected by companies allows these companies to accurately assess employees’ performance. I wished big data discussions were much more sophisticated and nuanced than that.
It is very hard to say if we find ourselves in an age of positivism again or not, because the term “positivism” is used in so many ways. If we agree that it basically equals empiricism, i.e. it refers to a technique of acquiring information through observation, in this sense I am proudly positivist. Or rather I try to be positivist. In reality it is extremely hard to forget internalized categories and look at data without presuppositions or bias. In the end, the most difficult thing is not data collecting or even learning how to do data science. The challenge lies in learning how to look at the results, because our thinking is so dependent on categories and values we had acquired previously.
It is also extremely difficult to be positivist when you study humans. I imagine it is way easier to analyse collections of atoms. Atoms don’t smile. But when you try to analyse photographs of smiling people, there is so much emotion at play that it makes it hard for scientist to be objective.
Have we already started defining ourselves differently as humans because of the amount of information we send every day?
Actually, we are not sharing that much information on social media. When you share a post with your friends, you don’t intend to share data or information with them. You share representations or captures of your experience. When you post a selfie, it’s not information, but an image. It only becomes data when business gets its hands on it. When business analyses how often you purchase products in an online bookstore, it is data. But when you share a post about the book you have just bought, you don’t produce data. You produce meanings, connections and you communicate. Data does not exist. We are only data for business.
As human beings, we are not very much different than we were 50 or 150 years ago. People still construct their lives in narratives. We frequently share more information than previously, but I’m not sure how important this quantitative increase is. The point is not that we are changing. The point is that we are not changing enough! If you want to be a twenty-first-century artist, please don’t make paintings, installations or public art projects, but change the human being. Make a human being, which would be more appropriate to the technology we have! Do not produce art for existing humans! The problem is artists don’t want to spend time on creating new ideas, they want to do business and sell well. It seems the only place you can find creativity these days is food.
Should we defend the place of liberal arts in our societies to preserve some remnants of creativity?
I am not going to extoll liberal arts, because I am myself a professor of computer science. I ran away! Obviously, we want people to know about the history of their societies. We want people in Poland to know the writings of the best Polish writers, but also those of Balzac and Dostoyevsky. We also want people to be able to communicate. And we want people to be able to look at each other as human beings, not data sets.
The point is that people who cannot add up numbers, or use social media, or do data science, are basically making themselves irrelevant. Humanities should become more aware of programming and new digital tools. Digital humanities is a very promising field. We need the humanities to become more data-friendly and a little bit more contemporary.
On the other hand, when we educate engineers, we put efficiency before all. When we give engineers data, they optimize them in order to make a better bridge and create a smart city. But human beings are not only about efficiency. When you work on a short essay, you don’t write it mechanically, but you suffer and invest your feelings in the process.
What we need is to modernize the humanities and humanize technology.